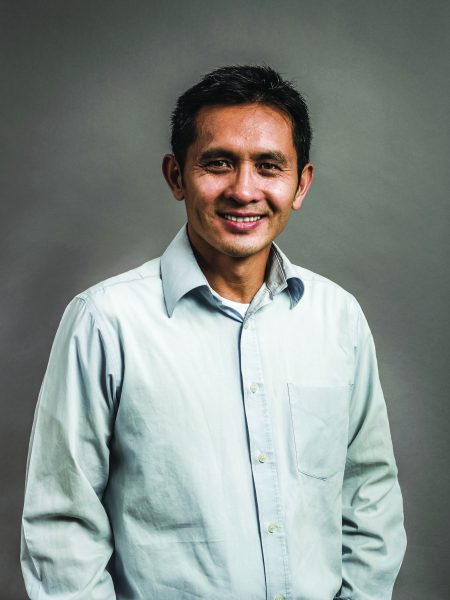
There are two positive trends in our industry that now need to come together. First, our industry has moved from the murky waters of fraud, unsafe content, auction tricks and a general opaqueness to a cleaner and more transparent ecosystem. To be clear, though, the work is not done. We are not yet staring at crystal clear, blue waters but it’s getting there. Second, the promise of artificial intelligence (AI) in advertising is starting to become more of a reality. We can predict outcomes better, target users more accurately and vary the ad creative we serve to users based on their profile. However, like any new toy, the hype is greater than the reality and everyone is claiming they are using AI and machine learning but almost no one is explaining exactly how they do it.
Like the trend towards transparency in almost everything else that is happening in the industry, we need to get rid of this black box. Why do ad tech companies build an AI black box? A valid reason is that the AI is proprietary and the company doesn’t want to give away its secret sauce. I find this explanation at best weak and at worse trying to hide the fact they don’t have AI. It is weak because explaining what you do in AI doesn’t mean you disclose every little bit of detail about the AI models you develop. You can explain a model and it doesn’t mean a competitor can easily copy it. They still need the know-how, the experience and the data needed to inform the model. But disclosing more of what you do leads to more credibility and more trust, which far outweighs the risks.
For many companies in our space, claiming AI is marketing spin. Not saying much beyond that is a way to hide the fact they are not doing much at all with AI. What they claim is AI is, in many cases, a lot of humans in the back doing the work. There may be some machine thinking, but it is mostly humans at work. When you look under the hood (if you are allowed), you will see that many machine learning algorithms are simply basic codes that do little to no machine learning at all. Is the company claiming that it uses machine learning to optimise towards better viewability actually doing this? Or is there a person involved in the optimisation? More importantly, does it matter if the results are good and the cost is of good value?
Yes, it matters. Why? Because if you then want to scale a successful test, how will the need for more human optimisation scale? If you want to optimise in real-time, a human cannot do this. If you want to optimise in multiple dimensions and improve your optimisation every day, a human cannot do this efficiently. So, in the end, a successful test campaign is just that: a test campaign that doesn’t necessarily repeat itself in a scaled environment.
Advertisers are now very suspicious of black boxes, so that in itself is another reason to demonstrate transparency and build trust with them.
As an advertiser, how can you determine if a company is really leveraging AI? Here are a few questions you can ask:
- What is your definition of AI and what type of AI do you use? I find that most companies that use the term AI without further explanation are simply not doing much with AI.
If a company replies they are using machine learning, this is a better answer. Most examples of AI in advertising are machine learning, which is a type of AI where the system ingests data to make a prediction (e.g. to predict if this ad impression will be viewable) and learns from results and further data to improve the prediction over time. - Can you explain how your machine learning works? The company doesn’t need to open the kimono more to give away all its secrets, but it can explain an overview of the model. For example, to predict whether an ad will be viewable, the platform ingests data.
- Can you provide a case study that proves that the machine learning is producing superior results over a control case where the machine learning was not deployed? Can you show how this was then scaled and still produced results? This forces the company to prove to you it works, not just in a small test campaign.
- Can your machine learning optimise more than one KPI at the same time? Many times, companies simply optimise towards one KPI, which is machine learning but is fairly rudimentary, and in some cases is not more efficient than if humans
did the work. When a company can optimise towards multiple KPIs, then it shows it can outperform humans. - How often are the models updated? If the models are updated hourly or daily then they are strong. If the models are updated less frequently, than it’s not much better than human learning and we as humans are slow learners.
Let’s challenge those who claim AI and let’s arm advertisers with ways to sniff out those who are over-selling. Then AI can be seen in our industry as a revolutionary tool to deliver better results more efficiently, and not just a buzzword.